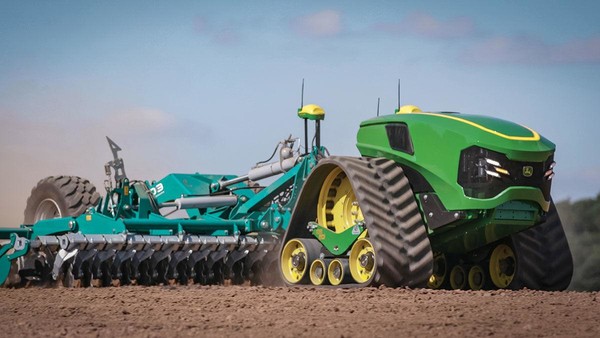
Agricultural machinery: the age of robots
Electronic systems are now growing exponentially, and the formidable spread of digital products offers particularly beneficial economies of scale. This has very important effects on agriculture "4.0" and on the application of robotics and artificial intelligence in the agricultural machinery sector. What are the limits within which machines can learn and operate, and how can they test their behaviours, in particular when they have to do with human interaction: this is the issue that the sector's industries are already taking on
The issue of robotics is one of the technological pillars of 2020 and the years to come. And with good reason. In recent years, there has never been such an effort on this front at a global level and in every industrial category, and there are many reasons for this. First of all, the costs: no technology, however innovative, can have a major impact on industry and consumers if it is not affordable and profitable. Some will remember Moore's Law, which between the 60s and 70s argued, perhaps in a visionary way, that "The complexity of a microcircuit, measured for example by the number of transistors per chip, doubles every 18 months (and quadruples therefore every 3 years)." This assumption, which can be put in more or less direct relation with the microcircuit's computing power, has proven correct for decades... until now.
Today it is no longer true. Today's electronic systems are produced with nanometric technologies used for increasingly advanced silicon manufacturing processes, and these have led to a reduction in the size of transistor gates to today's limit of 5nm. This has an important effect on the behaviour of the systems, since approaching its physical limits means silicon stops having a linear behaviour, thus affecting the behaviour and performance of the chips. Then there was the work on the chip architectures, communication buses and a number of other factors that led to the processors' computing power no longer growing linearly with their complexity, but rather in a way similar to an exponential curve. It is clear that the spread of smartphones, smart-tvs, smartwatches, etc., in the consumer market has led to a veritable technological war that has increased the speed in the development of increasingly high-performing microcontrollers at ever lower costs, as they are linked to an economy of increasing scale from year to year, if not from month to month. This, of course, has an impact on the computing power and costs of the systems used on agricultural machines.
In light of this, it is clear that many manufacturers discovered the possibility of providing new services to their customers, making engineering efforts in order to make their machines more efficient by "making them think." By now we all have a mobile phone with an AI-equipped camera (Artificial Intelligence) that adapts to the shooting conditions. We all have a TV with algorithms that adapt the image quality, colors or brightness to the content or environment where you are watching your favourite movie. Therefore, it is not so hard to think that agricultural machines could also adapt their operation to a series of parameters detected in real time directly in the field. This is what the above manufacturers asked themselves, striving to figure out how to make their workflow more efficient and adaptive to situations. And the entry barrier due to the cost of access to technology has been greatly reduced, if not almost completely eliminated. This provided the first decisive thrust, not forgetting the growing accessibility to increasingly complex sensors capable of detecting features that were unthinkable until a few years ago, such as the amount of chlorophyll in a leaf or the level of nutrients in a fruit.
However, there was another very important contribution to research towards technological innovation: the European Green Deal! A concrete European commitment to a cleaner world and environmentally friendly practices: a very important issue in an era when we are ravaging our planet. Whether we accept it or not, agricultural practices have an important impact in all this, not to mention the need to meet the challenges linked to the world's increasing population and the resulting need to produce more and better, wasting less and preserving the environment. And in all of this, technology has the lion's share. It allows us to avoid waste, applying treatments only where it is actually needed, using water resources rationally, and optimizing the working time and distance travelled by the machines, thus reducing consumption and, ultimately, perform every step while adapting precisely to the crop's conditions. A concrete case? Suppose there is an infestation of our field or our crop. Normally we would hook up our sprayer to the tractor and start "opening fire" or, in other words, opening the nozzles and spreading pesticide. But what if only a few plants scattered here and there had been infected? We would have wasted a huge amount of raw material (which costs dearly), we would have gone further than we should with the machines, wasting fuel (also expensive), and we would have wasted time and polluted the crop, the soil and the water resources, with considerable impacts on our health and the environment in which we live. Of course, with precision farming technologies we would have improved the situation a lot already, but we still would not have come to the optimal solution. However, if we had a system with a high-resolution (4K for example) camera or other, a processing system equipped with appropriate Artificial Intelligence algorithms could analyse the captured images in order to identify pathogens and, only in in this case and for the plant under consideration, activate the treatment by modifying the doses on the basis of, for example, the density of leaves, wind and humidity. It seems almost pointless to list the purely economic and environmental benefits of such an operation. Several studies have shown that cost reduction per hectare can exceed 60% depending on the type of operation. Science fiction? No! There are already machines that do this, because for years the technologies have been mature, and now they are accessible. Some companies are working to make such machines fully autonomous, so they do not need to be driven and operated by a human being, who can then sit comfortably at home to control the operations remotely.
"That is all well and good - some may say - but today I still have to go in the field with my machine and drive all over its surface, even if the machine then operates only where needed." This is not the case because with a drone, for example, is possible identify the infected macro-areas from above using its sensors, then go into the field to operate only in those areas with targeted plant-by-plant treatment. We could give a multitude of other concrete examples ranging from soil processing to fruit and vegetable harvesting and haymaking machines. In short, it is no coincidence that robotics, understood in its broadest sense, is thus at the heart of European strategies, particularly in our field. It is also no coincidence that almost half of Europe's agri-food - if not specifically agricultural - projects under Horizon 2020 are related to robotics. And probably the other half regards big data and connectivity, because even the most complex machine can do little if it's not integrated into a larger virtuous system. Also, as part of CEMA (the European Association of Agricultural Machine Manufacturers) there are two specific working groups on autonomous machine capabilities and artificial intelligence. A third group, focused on precision agriculture, is converging more and more on the themes of the first two and on the topic of connectivity. This is because it is essential that the directives and requirements that will regulate the technological contents of agricultural machinery in the near future are discussed and proposed by those who have the necessary knowledge and expertise in this field. It is necessary to raise the awareness of the European Commission about these issues by making concrete contributions based as much as possible on direct experience. We could talk at length about how and when we are discussing these issues and what proposals have been put forward so far, but perhaps it is best to delve into the matter when there will be less confusion, given the size and complexity of the issues. Returning to the central topic, someone may have some doubts after what they have read so far: "are you talking about robotics or Artificial Intelligence?" Actually, we are talking about both and more.
An automated agricultural machine is programmed to perform certain tasks. Certain parameters can be detected through sensors in order to modify the processing, but this is performed in a predetermined manner by static decision-making algorithms. At best this machine can be a robot, able to move and operate autonomously and where human intervention is necessary only in emergency situations, but with a behaviour that is always based on known algorithms. We could even consider the machine dumb, albeit much more efficient than a normal agricultural machine for the reasons we illustrated above. However, today such machines and systems are becoming integrated, thanks to the available computing power and soon thanks to cloud computing. These new strategies that encroach on artificial intelligence and make (or can make) machines capable of learning and adapting to the most varied situations, even if not known in advance. We no longer have to deal with algorithms that flow down a decision-making tree until they arrive at one of the predetermined solutions, but with decision-making patterns that evolve by integrating new cases as the machine faces new situations. Its first response may be incorrect, but the system can assess the impact of its choice and adapt the decision-making pattern to improve the result time after time. Or a system can be trained, thus providing a basic decision-making pattern that can evolve through new "training" sessions, if that's what we want to call them. Moreover, the surface of artificial intelligence has barely been scratched, with an infinite number of facets ranging from machine learning to deep learning, from neural networks to fuzzy logic. It would take stacks of books to analyse these technologies, and we certainly do not aim to indoctrinate anyone through a popular article like this.
It is important, however, to understand how much these technologies are increasingly entering our daily personal and working lives, interacting with each of our choices or making decisions in our place, and how important all this can be when it comes to agricultural tasks. Here is the core issue that will arise in the near future and which is already being debated today: what are the limits within which machines can learn? What are the limits within which it is safe to operate them and, above all, how can they test and validate their decisions and behaviours, especially when they have to do with human interaction? These are the questions that the entire industry of agricultural machinery manufacturers, supported by an increasing number of robotics and AI experts, is asking itself. It is impossible to fully predict how machines will react, but it is still necessary to define behavioural and decision-making limits that can be verified and that ensure safety in their daily use.